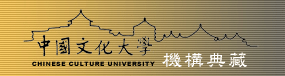 |
English
|
正體中文
|
简体中文
|
全文筆數/總筆數 : 47184/51050 (92%)
造訪人次 : 13925306
線上人數 : 365
|
|
|
資料載入中.....
|
請使用永久網址來引用或連結此文件:
https://irlib.pccu.edu.tw/handle/987654321/28352
|
題名: | 應用約略集合理論、決策樹、貝氏網路與類神經網路預測企業財務危機 Applying Rough Set Theory, Decision Tree, Bayesian Network,and Artificial Neural Network for Predicting Financial Distress of Enterprises |
作者: | 謝孟儒 Xie, Meng-Ru |
貢獻者: | 會計學系 |
關鍵詞: | 財務危機 資料探勘 約略集合 決策樹 Financial Distress Data Mining Rough Sets Theory Decision Tree |
日期: | 2014-06 |
上傳時間: | 2014-09-30 17:57:17 (UTC+8) |
摘要: | 以往對預測企業財務危機都是用傳統的迴歸模式,最近有許多學者使用資料探勘方法針對預測企業財務危機做研究,準確度有提高,但整體文獻上還不夠完整,所以本研究以資料探勘預測企業財務危機,希望能得出一個更準確地偵測模式。本研究應用2004年到2013年發生財務危機公司當作樣本以一家財務危機公司對三家財務正常公司的方式進行比對,嘗試以約略集合理論、決策樹QUEST與貝氏網路結合較新之人工智慧演算法「決策樹 C5.0」、「類神經網路」來建立一個二階段的財務危機預警模型,並探討不同變數篩選方法與決策樹 C5.0和類神經網路所建立之模型效率差異。本研究發現,以決策樹QUEST來進行變數篩選結合決策樹 C5.0將有助於提升模型整體準確率,準確率達95.45%型一的錯誤率為6.67%較類神經網路優越,本研究結果顯示以決策樹QUEST篩選搭配決策樹 C5.0可以有效地預測企業財務危機。
In the past, to predict corporate financial distress was using traditional regression model. Recently, many scholars have been using data mining methods to predict corporate financial distress and the accuracy has improved. However, the overall lit-erature is not complete. Therefore, this study uses data mining to predict corporate financial distress so as to achieve a more accurate detection mode. In this study, the samples are selected from financial distress companies between 2004 and 2013. The approach is using a sample of financial distress company to the three normal com-pa-nies as a comparison. It tries using rough set theory, decision trees, QUEST, and Bayesian networks to combine with the relatively new artificial intelligence al-go-rithms C5.0 Decision Tree, neural network for creating a two stage financial dis-tress prediction model. Moreover, this study also explores different variables screen-ing methods, C5.0 decision tree and neural network to establish a model of effi-ciency difference. This study found that the decision trees, QUEST, to combine variable screening C5.0 decision tree will helpful to improve the model’s overall ac-curacy. The accuracy rate is 95.45% and error rate of type 1 is 6.67%, which superior to the neural network. The results show that the decision trees, QUEST, screening with C5.0 decision tree can effectively predict corporate financial distress. |
顯示於類別: | [會計學系暨研究所 ] 博碩士論文
|
在CCUR中所有的資料項目都受到原著作權保護.
|